The following end of contract report was delivered to Manatū Hauora Ministry of Health at the end of August 2023. It covers all activities of the final year of the Covid-19 Modelling Aotearoa programme, with an additional, more comprehensive bibliography including all publications since the start of the programme in 2020. You can download the report as a PDF, or click on each heading below.
![]() Professor Michael Plank |
![]() Dr Dion O'Neale |
Looking back over the last three and half years, it is truly incredible to see the breadth and sheer volume of mathematical and computational modelling work that has been done by the dedicated group of researchers at Covid-19 Modelling Aotearoa (CMA). The impact of this work ranged from warning decision-makers about the scale of what we were facing in March 2020, supporting the elimination strategy and vaccine rollout through 2020-21, to informing decisions about mitigation measures and healthcare capacity planning in 2022-23.
This report primarily relates to the period covered by the most recent CMA contract with Manatū Hauora and the Department of Prime Minister and Cabinet (spanning July 2022 to June 2023) and the researchers involved during that time. However, we have also listed publications and software outputs from earlier periods where they relate to the CMA team from the most recent period.
We hope that by making these tools and reports more widely available, Aotearoa and further afield will be in a better position to respond to future outbreaks of infectious disease.
This work was only possible because people were willing to pivot their research activities and adapt to working in the pressurised and fast-moving environment of an emergency response. We feel very proud of what this team has achieved, and the support we have been able to provide to multiple aspects of New Zealand’s pandemic response.
It has been an immense privilege to work alongside the talented and hard-working researchers and professional staff in CMA, as well as our dedicated colleagues in central government and the national health system. Huge thanks are due to everyone who has been involved, in big ways and small – what we’ve achieved would not have been possible without you.
Michael Plank is a Professor in the School of Mathematics and Statistics at the University of Canterbury, a Fellow of the New Zealand Mathematical Society, and an Investigator at Te Pūnaha Matatini.
Dr Dion O'Neale is a Senior Lecture in Physics in the Faculty of Science at the University of Auckland, and an investigator at Te Pūnaha Matatini.
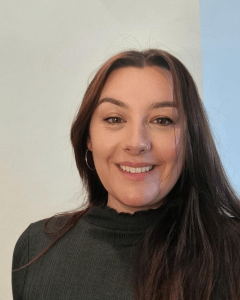
Victoria Louise Smith
When the pandemic hit our shores in 2020, researchers from across Aotearoa sprang into action to support efforts to prevent spread and minimise harm. Our group, now collectively known as COVID-19 Modelling Aotearoa (CMA), didn’t have a name then – but the drive to contribute to protecting Aotearoa from this new but very real threat brought us together.
Early on, our proposed research programme spanned a wide range of crucial areas, including modelling, ethics, phylodynamics, and communication. Our collaborative network quickly expanded to include over 80 individuals from more than 12 different organisations.
As the demands of the pandemic response evolved, so too did CMA. Our programme adapted to home in on infectious disease modelling, providing detailed insights into a range of important aspects, such as potential viral spread, variants of concern and vaccination uptake.
However, just like so many communities in Aotearoa during the pandemic, one constant remained throughout our journey: the tremendous dedication, effort and energy invested by every member of CMA over the course of our more than 3-year programme. For many of us, this commitment meant putting aside all other research pursuits, family time and sleep to fulfil urgent and critical work. It required adapting to new ways of working, delivering rapid and frequent reports to diverse audiences, effectively communicating complex data and issues to the public, working double-time and balancing multiple roles, and acquiring new knowledge and skillsets in tight timeframes.
I would sincerely like to acknowledge and thank the many researchers, students, professionals and collaborators who contributed tirelessly throughout the programme, whose mahi not only demonstrates the resilience of our communities but also exemplifies the real-world impact of our scientific endeavours.
Victoria Louise Smith is a Research Manager in the Department of Physics at the University of Auckland.

Associate Professor Matthew Parry
The plan for a peer review panel was first proposed on March 31, 2020 by Shaun Hendy. With COVID-19 modelling being carried out by his team increasingly underpinning ongoing advice to Government, Shaun realised that it was important to obtain independent review of the modelling. Since events were taking place too quickly for a formal peer review process, the proposal was for the formation of a diverse group of experts to provide rapid, as-required reviews. Matthew Parry, a principal investigator at Te Pūnaha Matatini, was approached to chair the panel. By April 7, 2020, the panel had been assembled for a funding application to MBIE, and the first review appeared on April 8, 2020.
In addition to a focus on technical modelling issues, the importance of equity issues was also identified from the outset. For example, “what questions should modelling be addressing?” were also considered in-scope. Indeed, the very first report identified the need for an age-structured model of Covid transmission, as well as querying whether parametric uncertainty had been adequately accounted for in model predictions.
To answer the variety of questions posed of it, the panel originally comprised Samik Datta (epidemiology and modelling), Nigel French (epidemiology and modelling), Markus Luczak-Roesch (modelling and computing), Melissa McLeod (Māori health and public health), Anja Mizdrak (public health), and Fraser Morgan (agent-based modelling). The members were approached based on contacts within Te Pūnaha Matatini or on the recommendation of members of the COVID-19 Technical Advisory Group. The inclusion of Fraser was in recognition, even in April 2020, that an agent-based model or network model would likely be required.
In 2022, Samik moved to the modelling team, and the panel was expanded to include Amanda Kvalsvig (public health), Linda Martis (data science), Patricia Priest (epidemiology) and Ben Ritchie (data science). The panel also co-opted Thomas Lumley (survey statistics), Patrick Walsh (economics) and Maksym Polyakov (economics) for one-off advice.
Each review was initiated by a draft report sent from the modelling team, usually with a timeline attached. The report was then sent on to the panel members and a Zoom meeting scheduled. After the meeting, the chair typed up the notes and shared the review simultaneously with panel members and the modelling team as “living document”. This enabled more immediate feedback and, when necessary, further discussion with the modelling team.
As the timeline below indicates, the peer review panel was not called upon uniformly in time. Not surprisingly, peak activity typically coincided with outbreaks or new variants of concern.
Number count of peer review panel reports in time
Ultimately, 36 reports were written by the peer review panel from April 2020 through to April 2023 – with a 37th snuck in after the end of the contract! Topics reviewed included the effect of different alert levels, elimination strategies, transmission within MIQ facilities, the effect of vaccination, variants of concern, and the impact of changes to isolation policies. One of the final reviews noted: “Working back from change in transmission to investigate scenarios of what caused the change is very nice. The approach taken is logical but it’s clearly challenging to draw strong conclusions for policy because of lack of validation data to narrow down the possibilities. It would be worthwhile to add a few bullet points about key information gaps and what would be needed to address them. Articulating this data need would make a strong argument for research explicitly designed to address information gaps, so future modelling can benefit.”
Matthew Parry is an Associate Professor in the Department of Mathematics and Statistics at the University of Otago and an Investigator with Te Pūnaha Matatini.
Management
- Professor Michael Plank, Programme Co-Lead, University of Auckland
- Dr Dion O’Neale, Programme Co-Lead, University of Auckland
- Victoria Louise Smith, Research Manager, University of Auckland
- Kylie Stewart, Communications Manager, Independent
- Matthew Mullin, Research Coordinator, University of Auckland
Compartment-based modelling
Professor Michael Plank, project lead, University of Canterbury
Michael led the development of a number of branching process and compartment-based mathematical models to address the ever-evolving disease transmission and policy environment in Aotearoa, ensuring that modelling outputs were policy-relevant and timely. In addition, Michael frequently appeared in news media to provide advice, explanation and commentary on COVID-19 for the New Zealand public. Michael is a professor in the School of Mathematics and Statistics at the University of Canterbury.
Dr Rachelle Binny, Manaaki Whenua
Rachelle contributed to the development and running the team's branching process model. She was also responsible for developing and running realtime reproduction number estimates from case data, and led the work that used New Zealand's detailed border testing data to get world-leading estimates of PCR sensitivity through time for SARS-CoV-2. Rachelle now works as a researcher in the wildlife ecology & management group at Manaaki Whenua Landcare Research.
Dr Samik Datta, NIWA
Samik developed pipelines and processes for automating the visualisation and communication of results from the compartment-based models. These approaches streamlined the presentation and interpretation of model outputs and made it easier to investigate larger numbers of scenarios when simulating multiple policy choices. Samik is a group manager for the Population Modelling team at NIWA.
Dr Audrey Lustig, Manaaki Whenua Landcare Research
Audrey led work investigating the potential impact of new variants of SARS-CoV-2 in New Zealand based on emerging international evidence. In addition, she contributed to the development and running of the team's branching process model and border risk assessment model, and co-ordinated the preparation of journal articles and reports.
Dr Oliver Maclaren, University of Auckland
Oliver contributed to the development of compartment-based models and refining model assumptions in light of changes in the situation on the ground. He provided valuable expertise in statistical methods for efficient parameter fitting and uncertainty quantification. This made it possible to better match the output of compartment-based models to changing data for case numbers,hospitalisations and deaths, and to effectively communicate model uncertainty. Oliver is a lecturer in the Engineering Science Department at the University of Auckland.
Dr Giorgia Vattiato, University of Canterbury
Georgia was responsible for ongoing simulation and development of the compartment-based modelling methods and code. This included ensuring that model parameters accurately reflected the situation on the ground, with an evolving pathogen and a changing transmission environment. Giorgia now works as a quantitative wildlife ecologist at Manaaki Whenua Landcare Research.
Dr Leighton Watson, University of Canterbury
Leighton developed a mathematical approach for estimating trends in Covid-19 infection numbers from wastewater data collected by ESR. This method also made it possible to infer relative changes in the effective reproduction number and in the case ascertainment rate as testing and reporting behaviour changed over time. Leighton is a lecturer in the School of Mathematics and Statistics at the University of Canterbury.
Network- and individual-based modelling
Dr Dion O’Neale, project co-lead, University of Auckland
Dion co-led the network- and individual-based modelling team. Dion was a strong advocate for the importance of considering individual and community heterogeneity when modelling disease and policy impacts. In particular, Dion spearheaded the development of the bipartite Populated Aotearoa Interaction Network (PAIN), in order to be able to simulate of spread of COVID-19 more realistically, and to better address equity implications of mathematical modelling. Dion also regularly responded to journalists to provide advice, explanation and commentary on COVID-19 for the NZ public. Dion is a senior lecturer in the Physics department at the University of Auckland.
Dr Emily Harvey, project co-lead, Market Economics
Emily co-led the network- and individual-based modelling team. In addition to coordinating, supervising, and contributing to the various projects and reports for the team, Emily's contribution also involved developing a number of new mathematical modelling and analysis approaches. Amongst these was a software package for analysis of longitudinal participatory surveillance data for estimating prevalence of respiratory disease in Aotearoa. Emily is a senior researcher at Market Economics.
Dr James Gilmour, University of Auckland
James was responsible for developing and updating the code for building the Populated Aotearoa Interaction Network (PAIN). He also wrote software for analysing the networks produced by PAIN and the contagion simulation results from CoBiN. James now works as an Actuarial consultant at EY.
Joshua Looker, University of Auckland
Josh developed, maintained, documented, and tested both the PAIN and CoBiN libraries, in addition to parametrising and running contagion simulations. He also led the work on characterising household secondary attack rates. Josh is now studying for a PhD in Applied Mathematics at the University of Warwick.
Dr Gray Manicom, University of Auckland
Gray was responsible for parametrising the effect of vaccination and immunity history in the contagion model, including testing and validation of the CoBiN library. Gray also contributed code for characterising community interactions the PAIN library and investigated the effects of heterogeneous vaccination coverage in the contagion modelling. When last we heard, Gray was driving across southern Africa on a multi-country safari before he moves on to the next step in his career.
Frankie Patten-Elliot, University of Auckland
Frankie's role involved setting up, running and post-processing contagion simulation results in a high-performance computing environment. He was responsible for ensuring that simulation scenarios were appropriately parameterised and that the output was analysed in preparation for reporting insights. Frankie also contributed extensively to the development and testing of the CoBiN library. Frankie is now studying for a PhD in Mathematical Biology at the University of Nottingham
Ella Priest Forsyth, Market Economics
Ella's contributions spanned developing and testing code, managing simulation and analysis pipelines, authoring reports, and presenting results to stakeholders. As part of this, Ella developed new analytic approaches for processing contagion simulation results and for updating model parameters to fit with an evolving pathogen and an changing environment of transmission control measures. We're hoping that we've convinced Ella that doing a PhD about contagion on networks would be a fulfilling next step in her career.
Joel Trent, University of Auckland
Joel led the development of code for stochastic simulations to quantify the effect of antigen tests for different isolation and quarantine scenarios. This led to the MitigatingIsolationandQuarantine.jl library, the teams only library in the Julia programming language, and probably the best documented. Joel is now a graduate reservoir engineer with Contact Energy.
Dr Steven Turnbull, University of Auckland
Steven was responsible for compiling and analysing the demographic information necessary for building a synthetic population for Aotearoa using the PAIN library. He also developed a number of R Shiny applications for communication and visualisation of data, including vaccination rates and distribution of transmission risk and vulnerability. Steven is now a senior data analyst at Synergia Consulting.
Dr David Wu, University of Auckland
David led the development of the code for stochastic simulations of Contagion on a Bipartite Network — the CoBiN library. He also contributed expertise with software engineering practices, helping to automate and proceduralise the team's work in a high performance computing environment. David is now a post-doc at Monash University in Australia.
Kylie Stewart, Communications Manager
Kylie is a science communicaton specialist based in Kirikiriroa whose previous clients have included The MacDiarmid Institute for Advanced Materials and Nanotechnology and Te Pūnaha Matatini. She acted as media liaison and internal and external communications manager for Covid-19 Modelling Aotearoa.
Epidemiology and Public Health
Professor Trish Priest, University of Otago
Patricia is a New Zealand public health scientist and epidemiologist who is Professor of Public Health in Medicine at the University of Otago. Throughout the COVID-19 pandemic, she served as an advisor to Covid-19 Modelling Aotearoa, and the New Zealand Ministry of Health.
Distinguished Professor Nigel French, Massey University
Nigel is Distinguished Professor of Infectious Disease Epidemiology and Public Health at Massey University, New Zealand. He is Chief Science Advisor for Te Niwha, the Infectious Diseases Research Platform, Executive Director of the Infectious Disease Research Centre and Emeritus Director of the New Zealand Food Safety Science and Research Centre. Nigel has worked on the epidemiology and control of infectious diseases of national and global importance. He served as an advisor to Covid-19 Modelling Aotearoa.
Mobility Modelling
- Aaron Cutter, Project Lead, Finity
- Jevon Fullbrook, Finity
- Emma Vitz, Finity
- Tyler Dent, Finity
Software automation and code review
- Pieta Brown, Precision Driven Health
- Ning Hua, Precision Driven Health
- Rachel Owens, Precision Driven Health
- Dr Kevin Ross, Precision Driven Health
Peer review
- Associate Professor Matthew Parry, Peer Review Lead, University of Otago
- Distinguished Professor Nigel French, Massey University
- Dr Amanda Kvalsvig, University of Otago
- Associate Professor Markus Luczak-Roesch, Victoria University of Wellington
- Dr Melissa McLeod, University of Otago
- Dr Anja Mizdrak, University of Otago
- Dr Fraser Morgan, Manaaki Whenua – Landcare Research
- Ben Richie, Nicholson Consulting
Covid-19 Modelling Aotearoa would like to acknowledge the contributions of numerous colleagues in previous iterations of the programme since 2020.
A mathematical model is a simplified representation of a real-world phenomenon expressed in mathematical language. In epidemiology, models are used to understand the way infectious diseases spread through populations. They combine basic principles of how a disease spreads – contact between someone who is infectious and someone who is susceptible – with characteristics of the population to estimate how many people will get infected over time.
Mathematical modelling is a powerful tool for supporting impact assessment and planning, interpreting raw epidemiological and clinical data streams, providing situational awareness, evaluating control measure effectiveness, and comparing alternative policy options. Models provide a framework to think systematically about the consequences of a range of assumptions, and test which assumptions are consistent with empirical data.
Models typically have inputs relating to:
- Characteristics of the pathogen, such as the incubation period, infectiousness, and age-specific risk of symptoms, hospitalisation or death.
- Characteristics of the population, such as the age structure, ethnicity, and the level of immunity either from vaccination or from previous infection.
- Some estimates of the rates of contact between people, including interaction patterns and social structure, and how these are affected by various public health interventions.
Some of these inputs are taken directly from real data. Others cannot be observed directly or are uncertain and so assumptions need to be made. Models are typically run for a range of different assumptions to investigate how much this affects key outcomes. Models are often developed iteratively, by comparing model outputs to new data and refining assumptions as needed. This process itself can help identify areas of uncertainty or important drivers of outcomes.
All models are a simplification of reality. They cannot capture every detail about who is interacting with whom, how that changes over time, and the myriad of variations between individuals. Instead, they try to focus on the most important factors that affect the epidemic trajectory and its impacts at the population level. Deciding which simplifications and assumptions it is appropriate to use with a particular model depends on questions that you want the model to be able to answer. Sometimes the decision about which details to include depends on whether sufficient quality data is available about that variable, and whether it is likely to be important for the outcomes that are of interest.
Models are used in different ways depending on what scientists, health officials, or policy makers are trying to understand. Models can take recent data on cases to produce a short-term prediction (typically a few weeks), which may be useful for planning health care capacity. Models can be used to make medium-term projections (typically 2-3 months) of the epidemic trajectory if conditions stay as they are, or change in some specified way like relaxing public health measures. Models can also be used to investigate longer-term scenarios. These can be useful to think about “what if” type questions, like what if a new variant with specific characteristics emerges, but by necessity these include more uncertainty.
Governments may use modelling results to help inform decisions about policy. However, models are not the only factor considered and decisions are based on a range of data, evidence, and expertise. A model on its own cannot tell you what to do, but it can help weigh up the pros and cons of alternative options.
Ordinary Differential Equation Model
A major component of Covid-19 Modelling Aotearoa’s work in 2022-23 was to develop, deploy and refine a compartment-based ordinary differential equation (ODE) model of Covid-19. This model was developed from the stochastic branching process model that had been developed between March 2020 and June 2022. The new model was motivated by the need for a more computationally efficient model suited to the large case numbers experienced with the Omicron variant, with reduced need to model stochastic effects and intensive case finding and contact tracing measures that were previously important when case numbers were lower.
Like the branching process model, the ODE model is age-structured and includes vaccination status, waning of vaccine-derived and infection-derived immunity, and time-varying contact patterns. The computational efficiency of the ODE model enabled a more rigorous approach to fitting the model to data on reported cases, hospital admissions and deaths caused by Covid-19.
The branching process model had previously been used to model the impact of potential new variants of SARS-CoV-2, results which informed the Ministry of Health’s Strategic Framework for COVID-19 Variants of Concern. In June 2022, whole genome sequencing data from ESR was used to estimate the growth advantage of the BA.5 variant over the previous BA.2 variant. This was a crucial model input in estimating the size of the BA.5 wave in winter 2022 [9]. Over time, additional features were added to the ODE model including rollouts of additional booster doses, policy changes such as the end of the Covid-19 Protection Framework in September 2022, reduced testing rates over time, seasonality, and the effect of antiviral medicines on fatality rates [58].
The ODE model was frequently used to give insight into the potential impact of policy changes, for example on mask use and case isolation requirements [28-30]. Estimates for the effect of alternative policy options on transmission rates were made using the network contagion model and/or the simulation model for isolation periods. The ODE model was also used to assess likely future demand for hospital-level care, particularly during winter.
Code supporting the models detailed in our published papers is available in public repositories – see individual papers for links.
Network Contagion Model
Most traditional models of disease spread, such as the compart-based models used by CMA, rely on a simplification called the “well mixed population assumption”. This assumes that individuals in the model interact stochastically, essentially bumping into (and infecting each other) at random. In contrast, a contagion network model assumes that people have specific interactions with other individuals and that infections can only take place via those interactions. A network contagion model is therefore well suited to modelling demographic effects (e.g. relationships between ethnicity and household size or industry sector for workers) and can explicitly represent the contexts where infections take place (e.g. infections acquired at home vs at work, school, or in the community). This additional complexity and more accurate representation of a population comes at the prices of greater computational cost and model complexity.
CMA developed an Aotearoa-specific network contagion model (NCM) in order to be able to better address equity concerns from disease spread and to better model the effect of specific scenarios such as changes to case isolation or contact quarantine rules where spread within dwellings affects disease dynamics.
The network: At the heart of the network contagion model is a detailed interaction network of approximately 5 million individuals in Aotearoa New Zealand. Each individual has the demographic characteristics of age, sex, ethnicity, and geographic location (Statistical Area – SA2) of usual-residence. Each individual also has a vaccination history. Individuals in the network are linked to specific group nodes or interaction contexts. These consist of dwellings, workplaces, educational institutions, and “community” events which cover all remaining interactions (e.g. sports, religious, social, & shopping interactions).
Dwellings are derived from Census data and reproduce the size and composition (age and ethnicity structure) observed in each SA2. Individuals in dwellings are linked to education and/or workplace nodes using education and workforce status and commuting data from census.
Workplaces and schools, within each SA2, reproduce age, ethnicity, and sex composition based on school roll and tax records. Schools are split into early childhood education, primary, and secondary. Workplaces have attributes of industry sector (level 1 ANZSIC06) and whether or not they have workers operating on site at various Alert Levels or Covid-19 Protection Framework (CPF) settings.
Community events have composition and size distributions drawn from the social networks literature, including factors such as ethnicity assortativity. Individuals attend events that are either short-range (near where they live, work, or study) or long-range (outside of their Territorial Authority, TA, of usual-residence). Patterns of long-range connections are determined for each SA2 using anonymised electronic transaction data from 2019 and 2020.
The contagion: Infection on the interaction network spreads via a stochastic contagion process. Infected individuals can, at random, transmit infection to those individuals to whom they are connected in the interaction network. If the transmission is successful, the new individual moves into an exposed state. Pathogen-specific parameters control the amount of time that infected individuals spend in the various disease progression states and the rate at which state transitions, including rate of infection, occur. These states include exposed, infectious (including asymptomatic), hospitalized, recovered, among others.
Most of the disease states can take the form of being unconfirmed, confirmed, and quarantined (or isolating). Subsequent state transitions depend on an individual’s current state. E.g. individuals who are isolating can only infect susceptible individuals from the same dwelling; individuals can only become hospitalized, or enter critical care, if their infection is symptomatic.
Disease transmission probabilities are affected by individual attributes including the vaccination status of individuals. Each individual is assigned a vaccination history with this record being derived from individual vaccination data according to age, ethnicity, and location (SA2).
The model: The model explicitly represents processes such as testing, tracing, and isolating, allowing these to be adjusted to reproduce specific Test, Trace, and Isolate (TTI) policies.
Individuals in the model seek testing at specified rates depending on whether or not they have symptoms, or are a known contact of a confirmed case. Test return rates are calibrated to published testing data.
Contact tracing processes in the model can be specified to follow different tracing processes and policies (with different parameters) for different interaction contexts. This can, for example, represent a policy where workplace contacts are only advised to seek a test if symptomatic, but interactions via another context are contacted by health officials and required to test and or isolate. Similarly, different isolation policies can be represented explicitly for different exposure contexts.
In addition to vaccination status, a range of non-pharmaceutical interventions can be represented, either directly or by proxy through additional processes. E.g. mask requirements reducing transmission in workplaces or schools at different CPF settings; closing specific interaction contexts (e.g. different industry sectors, schools); or restricting certain interaction contexts to only individuals with a specified vaccination status (e.g. vaccine pass requirements in hospitality settings).
Benefits of a network contagion model: Accurate network contagion models have significant extra complexity and overhead, compared with traditional contagion models. However, they have a number of features that make them attractive.
Most importantly, infection spread on networks has been shown to naturally capture contagion dynamics much more accurately than traditional well mixed models. One reason for this is that network models automatically capture the effects of local saturation.. In a well-mixed model, the number of people an infected person transmits the disease to is determined by the proportion of the overall population that is susceptible at that point in time. In contrast, individuals in a network model can only infect those susceptible individuals that they interact with during the period when they are infectious. For example, if all the contacts of an infectious individual in a network model have already been infected, that individual will not lead to any further infections, even if a large number of susceptible non-contacts exist in the overall population. This means that network models can separately capture the effects of individuals changing their interaction patterns, alongside the effect of other transmission reduction measures.
Network contagion models are well suited to capturing the effect of heterogeneity, both for individuals and in the effect of disease reduction interventions. As an example, simulations that apply a blanket transmission reduction of 50% to all workplaces display very different dynamics to simulations that apply a complete transmission reduction to 50% of workplaces and no reduction to the remaining 50%. When the former may show a reproduction number below 1 with infections dropping, the latter will show a reproduction number appreciably greater than 1, with infections growing.
A contagion network model is also able to capture effects that arise due to the correlation of factors. For example, several effects that increase transmission risk are known to be correlated. E.g. lower paid workers are less likely to be able to work from home, less likely to be able to isolate effectively, and more likely to live in larger households.
While any model is only as good as its assumptions and its input data, network models of disease contagion give the opportunity to simulate detailed scenarios. This is due not only to their natural suitability for including specific attributes of individuals, their interaction patterns, and the nature of theory interaction contexts, but also their ability to allow for detailed mechanistic processes (such as TTI policies and NPIs) that can take place on interaction networks.
FluTracking Analysis and Dashboard
During the elimination phase of the COVID-19 pandemic response, researchers at Covid-19 Modelling Aotearoa developed a statistical package for estimating the incidence of respiratory illnesses such as influenza, colds, and COVID-19 from the Flutracking participatory surveillance survey. Outputs from this analysis were initially used to estimate the number of tests needed for effective symptomatic testing coverage and for calculating the probability of detecting a community case of COVID-19 as symptomatic test-seeking behaviour from week to week.
With the move from an elimination strategy to a suppression approach, the outputs of the Flutracking analysis became an important source of information about the rates of spread of respiratory illness in Aotearoa. With increasingly uncertain case ascertainment rates, respiratory symptom incidence rates provided a useful sense check for plausible bounds on changing infection numbers over time, in different regions and age groups.
The CMA team also developed a public facing dashboard for communicating symptom incidence rates, including uncertainty estimates that responded to changing reporting rates. With help from Orion/PDH this dashboard has been re-published as SIICC – Symptom Incidence of Influenza, Cold, and Covid (https://siicc.covid19modelling.ac.nz).
Analysis of the Flutracking data required developing tools including de-biasing response data, population re-weighting, and calculating confidence intervals. The code for these has been published as a free-to-use, open-source software library. Methods from this library have since been adopted by ESR for their regular reporting of Flutracking survey results.
Stochastic Model for Isolation and Quarantine Testing
Investigators in CMA developed a stochastic simulation tool for calculating the likely effects of different policies for case isolation and contact quarantine.
Given a specific scenario for case isolation, or contact quarantine rules, these simulations were used to estimate metrics such as the average number of hours when a case would be infectious in the community post-release, the average hours of excess isolation when cases would be required to isolate beyond the end of their infectious period, and the proportion of infected contacts who would be detected. The CMA team used surveys of the evolving international literature on viral load for SARS-CoV-2 infections, and sensitivity of rapid antigen tests to accurately parameterise the evolution of infectivity for cases and the probability of testing positive on an antigen test.
Working in collaboration with analysts at NZ MoH, CMA researchers used case confirmation data from household contacts to infer test timing and to evaluate the efficacy of different quarantine policies with realistic parameterisation of the behaviour of contacts.
Simulation of different case isolation policy options was used to provide advice on how isolation policies could be chosen to balance cost or inconvenience against the risk of increasing infections in the community. These scenarios included quantifying the benefits of “test-to-release” for ending isolation policies and the effect of changing the start of the isolation period from symptom onset to test report date. See [37] for more details.
In addition to calculating the direct impact of policies, the stochastic simulation of isolation and quarantine library was used to calculate the necessary inputs for models such as CMAs ODE model for onward transmission of COVID-19 in order to estimate the future impact on case numbers from changes in case isolation policy. The code developed by CMA has been published as a fully documented and free to use open-source library `MitigatingIsolationAndQuarantine.jl` (https://cma-public-projects.gitlab.io/MitigatingIsolationAndQuarantine.jl/).
The Aotearoa Co-Incidence Network
Covid-19 Modelling Aotearoa researchers took advantage of Aotearoa’s unique linked data resource – the Integrated Data Infrastructure (IDI) – combined with novel network science approaches to build a tool that can estimate regions of high transmission risk based on interaction through work and education, without running contagion calculations. The Aotearoa Co-incidence Network, or ACN, uses administrative data for employment and education to build a network where households are linked if a member from each household is co-incident at the same workplace or education institution. These links between dwelling with co-incident individuals are then aggregated up to the SA2 spatial units (about the size of a suburb) before the resulting network of interactions between areas is analysed with a number of network science tools to infer measures like transmission risk and the geographic boundaries of connected communities.
By partnering with the GeoHealth Lab at the University of Canterbury, CMA researchers were able to publish a tool with interactive maps where estimated transmission risk could be viewed alongside areas with the highest vulnerability to severe consequences from a Covid-19 infection.
The ACN was subsequently extended, in joint work with analysts at the Ministry of Health, to include measles vaccination coverage. The resulting tool was used internally at MoH in responding to the emergence of measles in Aotearoa in the first half of 2023.
The source code for both the data analysis (to be run inside the IDI) and the visualisation tool (to produce interactive maps) have been made publicly available as an open source GitLab repository – see the software section of this report for details.
Wastewater Surveillance Modelling
Covid-19 Modelling Aotearoa researchers developed a new method for interpreting wastewater surveillance data in conjunction with data on reported cases of Covid-19. This method produces estimates of the time-varying reproduction number and relative changes in the case ascertainment rate over time. ESR funded additional work to operationalise this model on data from their wastewater sampling program and incorporate the results into their wastewater surveillance reporting to the Ministry of Health. See [26] for more details.
Te Whatu Ora Covid-19 Dashboard
Between February 2022 and June 2023, Covid-19 Modelling Aotearoa supplied results from the branching process model and later the ordinary differential equation model, which Te Whatu Ora (formerly TAS) displayed in a Power BI dashboard for DHB health planners. This allowed dashboard users to explore different scenarios for the future impact of Covid-19, for example showing the effect of different levels of behavioural change, variant impact, or seasonality. Model outputs for reported cases, hospital occupancy, and deaths were shown alongside data, which was updated in real-time. Te Whatu Ora disaggregated results from CMA’s national model to allow dashboard users to see information stratified by age and ethnicity, either nationally or in a selected Health Region or District. The results were accompanied by a description of the modelling assumptions, interpretation and limitations, co-designed by CMA and Te Whatu Ora. This was supported by verbal briefings to dashboard users by CMA researchers. Dashboard content was updated periodically to reflect changes in the epidemiological situation and updates to the model, for example to include the effect of reinfections, new variants and policy changes.
Covid-19 Forecasting Model
Covid-19 Modelling Aotearoa researchers developed a model to produce near-term forecasts (up to 3 weeks ahead) of Covid-19 cases and hospitalisations. This model is designed to be run in real time and be responsive to the latest trends in surveillance data. The model produces a quantitative forecast with associated uncertainty ranges to support healthcare planning. This is a different type of modelling approach to the ODE model, which is typically used for longer-term scenario analysis and comparison of alternative policy options. The forecasting model is more empirically driven and less mechanistic, meaning that it requires fewer assumptions and parameter estimates than the ODE model, but the results are only valid over a short time horizon.
The forecasting model has been operationalised in partnership with Precision Driven Health and Te Whatu Ora and provides weekly forecasts on real time data updates.
Through the University of Auckland, CMA contracted Finity Consulting Pty Ltd (Finity) to provide short term, geographically and demographically granular forecasts of COVID-19 infections and hospitalisations based on near real time mobility patterns in New Zealand. To meet this need, Finity produced a probabilistic model that combined a synthetic population dataset and mobile movement data to inform future spread of COVID- 19.
CMA researchers from the University of Auckland provided a synthetic population dataset, with one record for each individual in New Zealand. The dataset included:
- Household information
- Personal characteristics; Age/Gender/Ethnicit
- Employment status, type and location
- Education status and location
The model estimates future weeks’ cases and hospitalisations based on:
- Reported recent cases in the community, these are persons likely to infect or have already infected others they have interacted with
- Vaccination status and historical case history of individuals
- Transmission risk by location of infection outlined below
Transmission Location |
Effects Parameterised |
Home |
Number of people sharing a household, risk of others within the household having COVID-19 |
Work |
Location and type of work |
School |
Size of the school and risk of others within the same class having COVID-19 |
Community |
Informed by device level mobile movement data which was merged with the population dataset. If an individual either lives in or visits an area highly frequented by likely affected persons. |
Severity of infection parameters by age, gender and ethnicity were incorporated in projections of hospitalisation and death resulting from COVID-19.
The model was initially set with a number of model parameter values based on first-principles and literature review. A series of hyper parameter tunings were also run regularly to ensure that the models continued to reflect recent experience and therefore remain accurate for forth-coming weeks, but also to describe the changes in environment being observed.
The insights can be used to inform a variety of responses including estimating granular geographic case growth predictions, scenario testing movement restrictions, hospital capacity planning, population movement restrictions and compliance therewith and vaccination prioritisation.
Due to delays in the purchasing of the mobility data (Finity did not receive the mobile data until March of 2023), the mobility model was not used during the acute phases of the pandemic. In order to maximise the utility of the data and model developed, Finity presented COVID-19 use cases that were implemented for the New South Wales Ministry of Health and Victoria Department of Health in Australia and alternative use cases.
For the period 1 July 2022 to 30 June 2023, Orion Health subcontracted to the University of Auckland to provide model automation services under the COVID-19 Modelling Aotearoa (CMA) contract with the Department of Prime Minister and Cabinet (and later Te Whatu Ora) for real-time fitting and projection of epidemic dynamics including new infections, cases, hospitalisations and deaths at the national level.
Orion Health’s services were to involve:
- Leading the Orion Health team of Data Scientists
- Operationalising regular reporting of stable contagion model algorithms
- Performing routine data analysis as it relates to ongoing reporting
Specific contributions of the Orion Health team under this subcontract are summarised below.
Symptom Incidence of Influenza, Cold and COVID-19 (SIICC) Dashboard
The flutracker dashboard was a Shiny app developed by CMA to visualise flu survey data. Orion Health refactored the analysis code for this dashboard, created a React front-end for performance and scalability purposes, and deployed the dashboard on AWS. This dashboard is now called “Symptom Incidence of Influenza, Cold and COVID-19” or “SIICC” and can be found in the main menu at the top of this page.
Differential Equation Model
Orion Health initially investigated automating the ODE model however it was decided that this model would not be suitable for a high level of automation due to a requirement for oversight of scenario generation and outputs.
Instead, Orion Health conducted a peer review of the model code base, has documented this model, and has provided suggestions for code refactoring.
Covid Forecasting Model
The Covid Forecasting Model (CFM) provides nearer term forecasts for infections, hospitalisations, and deaths. Orion Health was contacted in late May 2023 by James Harris (from TAS, now Te Whatu Ora) with a request to automate this model so that model outputs were available over the winter months. Orion Health is in the process of completing this automation, hosted on AWS.
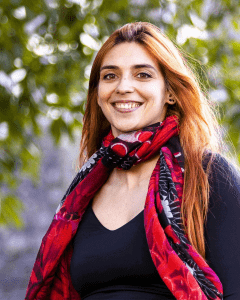
Dr Giorgia Vattiato
Georgia was responsible for ongoing simulation and development of the compartment-based modelling methods and code. This included ensuring that model parameters accurately reflected the situation on the ground, with an evolving pathogen and a changing transmission environment.
Covid-19 Modelling Aotearoa researcher Dr Giorgia Vattiato originally came to Aotearoa in 2018 for her PhD. “I grew up in Italy, studied engineering in France for 5 years and at the end of that time I decided I wanted to go into research and do a PhD. Specifically, I was looking for some modelling work that involved animal behaviour, and I found the perfect project with Te Pūnaha Matatini, in Christchurch, with Michael Plank, Rachelle Binny, Alex James, and Isabel Castro as my supervisors.”
Giorgia had just submitted her PhD thesis when she was asked to be part of the Covid-19 Modelling Aotearoa team by Michael Plank. As her supervisor, he knew she had some transferable skills that could be used to do the mahi, "although her PhD project was in a different area, I knew Giorgia had the excellent modelling and computational skills and the ability to put these to use in a new application area that were needed by the Covid-19 Modelling Aotearoa team, “Michael says.
Going from finishing a PhD to actively modelling a pandemic was challenging, and like many people, Giorgia had the added stress of not being able to travel to see her family. By the time she was finally able to return to Italy for a visit, she had gone nearly three years without seeing any member of her whānau.
“During the first year of COVID I was still in the middle of my PhD, and my supervisors and I had to move our weekly in-person meetings online. Work was slow, and it was sometimes frustrating not being able to exchange ideas around a physical whiteboard, but those online meetings kept the flow going and we made it work. During that time, I also used to hang out heaps with friends online; we kept each other supported.”
The tools Giorgia later used for Covid-19 Modelling were familiar, even though the work was quite different. “It was programming-based theoretical modelling, and that part was very familiar to me. The programming language was the same as the one I was using for my PhD. Everything else was new; I had very basic epidemiology knowledge so I had to ride quite a steep learning curve! The work rhythms were quite different, too: the CMA team had daily internal meetings, and weekly meetings with the government’s steering group. The day that I started with CMA was a government meeting day, and that was quite intimidating!”
Looking back on her time with CMA, there is one piece of work that stands out to Giorgia; “I was very happy with that first bit of work that we did when I started in 2021, when we were modelling the Traffic Light System. We were trying to simulate how long we would spend in each traffic light setting, and in different scenarios; it was really quite fun. I love creating cool visualizations and trying to explain science through plots and graphics, so I think that piece of work just tapped into my passion.”
Giorgia thinks now is a perfect time for Aotearoa to put in place a more formal pandemic preparedness plan. “This period where there's no emergency and no urgency to come out with models is the best time to develop them, so that when the next pandemic hits, we won’t have to rush as much. It's always risky, doing science fast. While our team had several great minds all working on a problem at the same time, it was still very a very high-pressure environment for everyone.”
Giorgia now works at Manaaki Whenua Landcare Research, where she is once again modelling wildlife populations with a focus on animal personalities and pest eradication. This might seem like a strange leap, but Giorgia explains: “the skills that I’m using are actually not that different than those from the CMA work. That’s the beauty of computational modelling: it’s always code on a screen, so if you like building models and programming, you’ll enjoy it regardless of what you’re applying it to.”
She looks back with pride on the mahi of CMA, “it felt a bit nostalgic when we had our last government meeting to hand over our model’s code. I think everybody should be so proud. I was thinking about how good and how important it was to have the diverse team that we had, and how much of a difference it made that everybody involved was able to contribute with a different set of eyes on the science we produced. The amount of knowledge and skills that were shared in our team was just amazing, and I think that everybody at CMA learned heaps in the last few years.”
Harvey, Emily P, Matt Hobbs, Joshua Looker, Dion R J O’Neale, and Steven Martin Turnbull. “Exploring COVID-19 Transmission Risk and Vulnerability Through the Aotearoa Co-Incidence Network (ACN).” Technical Report. Covid-19 Modelling Aotearoa, February 9, 2021.
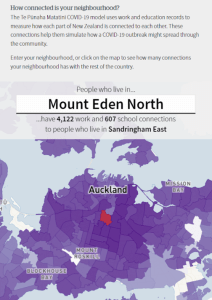
Screenshot from the NZ Herald, using our app to visualise transmission risk.
This research used data from Aotearoa New Zealand’s Integrated Data Infrastructure (IDI) to create a co-incidence network of workplace employment and school enrolment. The Aotearoa Co-incidence Network (ACN) provided a highly insightful tool to explore the manner in which the regions of Aotearoa New Zealand are connected to each other through co-incidence of individuals at workplaces and schools.
We showed how analysis of the network could be used to inform the (then) strategy of mitigating existing outbreaks (“stamp-it-out”) by revealing those sets of areas between which an outbreak is likely to spread most quickly. We also showed how analysis of the network structure can reveal spatially limited communities which can inform regional responses to disease outbreak (i.e., regional based interventions) should they need to occur, as well as specific areas of high transmission risk — both of these results could be used to aid a “prepare-for-it” strategy. Finally, we cross-referenced our findings with data on disease vulnerabilities (i.e., long-term health conditions, ethnicity, and deprivation) to highlight specific areas with a combination of high risk of contagious disease transmission and elevated vulnerability to such diseases.
We published an open-source library for the code and worked with MoH analysts to help them extend the code in the IDI to include early childhood education centres in the education interactions and to combine the co-incidence network with vaccination data. This went on to be used by Ministry of Health analysts in early 2023 as part of the response to the discovery of community cases of measles. A web-based app allowed for visualisation and exploration of transmission risk and vulnerability and was presented as a useful tool for decision and policy makers to inform more equitable responses to diseases such as COVID-19. It was also used to publicly communicate risk.
“Pick a neighbourhood in any New Zealand town or city, and then drop a single, hypothetical case of Covid-19 into it. Imagine that, from this one point, you could watch the coronavirus snake out into local schools, or into places where the adults living in that neighbourhood are likely to travel to and work.
Imagine that each of those connections goes on to create tens of thousands of new ones, which in turn create millions, as the outbreak spirals into a dense, spaghetti-like web of infection. Imagine that we could watch how this mind-bogglingly complex picture unfolds in real-time – and observe it dramatically change when we simulated a lockdown, or threw in the added power of testing and tracing.
This is just what scientists can now do, using a model so sophisticated and weighty that it requires a supercomputer to load and run.” – Jamie Morton, NZ Herald. September 6 2021.
Joshua Looker developed, maintained, documented, and tested both the PAIN and CoBiN libraries, in addition to parametrising and running contagion simulations. He also led the work on characterising household secondary attack rates.
Joshua came on board with Covid-19 Modelling Aotearoa (CMA) in mid 2021 while he was still working on an honours project supervised by CMA’s Dr Dion O’Neale, Dr Emily Harvey, and Dr Oliver McLaren. “At the start was I doing small tasks, just checking the model and stuff like that, but then very quickly into the new year, the Omicron wave hit and the work really started to ramp up, it was all hands on deck for everyone,” says Joshua. He admits at the time it was quite daunting, “but I preferred to just focus on the interesting maths side of it, and think about the long term implications of the work afterwards. It was really cool as an Early Career Researcher to see the immediate implications of the research… to see in real time just how much of an impact research can have.”
Though Joshua wasn’t one of the CMA team facing the media, he found himself being approached as an expert by people in his life, “suddenly most people that you know, know you’re working in Covid research, and they see you as the de facto expert. People began asking me if it was worth getting vaccinated, the sorts of questions more prominent researchers like Dion and Mike were being asked in the media, I was being asked by the people in my life.” A sentiment echoed by other members of the CMA team.
When asked to reflect on a piece of work he’s most proud of Joshua says, “I was in charge of leading the research work we did into characterizing secondary attack rates within different dwelling sizes, based on the algorithm choice that we used in our model. We haven't published that work yet, but it’s quite novel in that that we can’t really find any research anywhere else in the literature that dealt with the topic.”
He goes on to explain “We used something called a Frequency Dependent Model, which is where if you have more people in a dwelling, the chance that any particular individual will be infected goes down. Unfortunately, that doesn't quite model reality, in that larger, non-nuclear families, or multi-generational homes, and ethnicities associated with those types of dwellings, generally see higher attack rates. It was the opposite result from what our model predicted, and no-one in the existing literature has grappled with this. It was really nice to be put in charge of a novel research area into things that have actual equity implications in real life, especially if we were mis-modelling the attack rates within an already disadvantaged community.”
“Joshua made several important contributions to the individual and network-based modelling team” says CMA co-lead Dion O’Neale. “He was responsible for implementing a number of data processing pipelines and simplifying and automating various processes that were essential to the modelling and advice workflow. This helped to ensure that work was easily reproducible and verifiable, with fewer steps requiring tedious human intervention.
“Joshua’s work on improving the modelling of secondary attack rates for different dwelling sizes was another important contribution to the programme. Many traditional mathematical epidemiology models systematically underestimate the risk of secondary infections in larger dwellings. Although this is an easy problem to describe, the large number of different combinations that need to be considered to properly account for secondary infection pathways makes this a tricky problem mathematically. Joshua combined pen-and-paper analytic approaches with computational modelling in order to better characterise how household secondary attach rates change with household size.”
Joshua is now based in Warwickshire, England, where he is in the first year of a four-year PhD programme at the University of Warwick. His academic tutor, Professor Kat Rock is a mathematical epidemiologist interested in population-level disease dynamics, with a particular interest in vector-borne transmission and neglected tropical diseases. He hopes to continue working on epidemic modelling which addresses equity issues as he furthers his career.
Lustig, Audrey, Giorgia Vattiato, Oliver Maclaren, Leighton M. Watson, Samik Datta, and Michael J. Plank. “Modelling the Impact of the Omicron BA.5 Subvariant in New Zealand.”Journal of The Royal Society Interface 20, no. 199 (February 2023): 20220698.
Data and Matlab code to reproduce the analysis are available at https://github.com/michaelplanknz/modelling-ba5-in-nz
New Zealand experienced a wave of the Omicron variant of SARS-CoV-2 in early 2022, which occurred against a backdrop of high two-dose vaccination rates, ongoing roll-out of boosters and paediatric doses, and negligible levels of prior infection.
New Omicron subvariants have subsequently emerged with a significant growth advantage over the previously dominant BA.2. We investigated a mathematical model that included waning of vaccine-derived and infection-derived immunity, as well as the impact of the BA.5 subvariant which began spreading in New Zealand in May 2022. The model was used to provide scenarios to the New Zealand Government with differing levels of BA.5 growth advantage, helping to inform policy response and healthcare system preparedness during the winter period.
In all scenarios investigated, the projected peak in new infections during the BA.5 wave was smaller than in the first Omicron wave in March 2022. However, results indicated that the peak hospital occupancy was likely to be higher than in March 2022, primarily due to a shift in the age distribution of infections to older groups. We compare model results with subsequent epidemiological data and show that the model provided a good projection of cases, hospitalizations and deaths during the BA.5 wave.
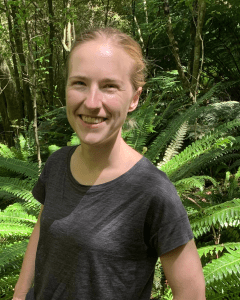
Ella Priest Forsyth
Ella Priest Forsyth’s contributions to the Network- and individual-based modelling team for Covid-19 Modelling Aotearoa (CMA) involved developing and testing code, managing simulation and analysis pipelines, authoring reports, and presenting results to stakeholders. As part of this, Ella developed new analytic approaches for processing contagion simulation results and for updating model parameters to fit with an evolving pathogen and a changing environment of transmission control measures.
We caught up with Ella from Copenhagen, where she was preparing to return to Aotearoa after a well-earned, post-CMA break in Europe. She originally travelled to attend The International Conference for Computational Social Science where Covid-19 Modelling Aotearoa researchers Dr Emily Harvey and Dr Dion O’Neale presented work the team had done.
Before Ella came to work with CMA, she had been working at the Ministry for Business and Innovation (MBIE) where she worked in Evidence and Insights in the energy and resources space. This work involved data collection, analysis, reporting and modelling, as well as work on statistic development. She approached Emily and Dion with a tentative interest in continuing her studies, perhaps with a PhD, and that’s how she came to join the CMA team in 2022.
“Ella was an unexpected lucky find for the CMA team,” said Dr Dion O’Neale. “She initially got in touch with us to talk about a possible PhD involving some modelling and analytics. James Gilmour was just about to leave the CMA team and Ella’s experience with combining technical work and clearly communicating results of that work made her the perfect addition to the team. Ella quickly became familiar with the complex models used in the individual- and network-based modelling team and led work on improving these and streamlining processes that helped to quickly produce quality assured, reproducible results.”
“Coming from a background working outside academia, including in engineering consultancies and government agencies, Ella brought with her a depth of experience about reporting to and for government as end-users and pragmatic project management expertise that is difficult to find in academic contexts. She also brought some tales of Tararua tramping to the team updates, providing the Auckland based team members with some vicarious wilderness experiences!”
Ella found there were some similarities with the modelling work she’d done at MBIE, but there were a lot of new things to learn, too. “At MBIE I did a lot of programming and working with models written in R (programming language) but the main model that that I worked with at CMA is written in Python, so it was similar to my work at MBIE but not,” Ella said. “The modelling that I was doing at MBIE was also obviously not looking at contagion on a network, so this was a great opportunity for me to broaden both my modelling and coding experience. I also used a High Performance Computer for the first time, so there were a number of cool new learning experiences that went with running the CMA model.”
When Ella joined the team, she immediately contributed to the weekly cross-government steering group meetings. “I found it stimulating and exciting because you were giving advice to the government, and often that advice would go on to become a news story. Timelines were often tight, but it was satisfying to think that the deadline was for someone to make a decision that was going to have an impact on the country.”
Ella says Emily and Dion are great managers and mentors. “They gave me opportunities to push outside of my comfort zone, things like presenting to the steering group in my first weeks of the job, but at the same time I felt supported. I did enjoy the fast-paced variety of the work and all of the opportunities for learning.”
Ella was also given opportunities on the international stage. She travelled to Turin, Italy with Emily to deliver a seminar at the Institute for Scientific Interchange. They presented on the Populated Aotearoa Interaction Network (PAIN) contagion model “Emily presented on the network and its construction, and I presented on the contagion model, it was a really, amazing opportunity.”
Ella believes that maintaining the networks, relationships and skills established during Covid-19 is crucial for any future pandemic preparedness.
“We need to continue to maintain those networks and modelling capability in a formal and transparent way, so that, if another pandemic were to hit, even if there's been no further work on it in the interim, there's a recognised network or task force of people who now are quite experienced and have relationships with each other.”
“It would be good to have some kind of formalised/published network that records people and their relevant experience, the people who could stand up a team, as well as the models that we have developed. I think it’s important to have some format that enables people with current experience AND people who develop experience in this area to be able to join and say “I'm here too and I'd like to be involved.”
She thinks there would be great value in publishing something reflective. “I know that the teams learned a lot over the last few years and having those lessons published somewhere would be valuable. These are the things that were great. These are the things we know now that we didn’t before. These are the mistakes we made, and in hindsight this is what we would have done differently.”
Ella is still not quite sure what the future holds, she’s going to take the summer to reflect on that, but while she hasn’t made her mind up just yet about that PhD, she does know that she will continue to work in modelling.
“I loved the research group atmosphere of coming with a problem to a meeting and bouncing things around and then going and trying new things… I left with a real appreciation of the breadth of the world of modelling. Not just contagion modelling, in this job I came into contact with lots of other modelling work. It did make me very enthusiastic for this area.”
O’Neale, Dion R J, Emily P Harvey, and Ella Priest-Forsyth. “Quantifying the Effect of a Change in Case Isolation Settings.” Covid-19 Modelling Aotearoa, April 20, 2023.
Harvey, Emily P, Joshua Looker, Dion R J O’Neale, Michael Plank, Ella Priest, and Joel Trent. “Quantifying the Impact of Isolation Period and the Use of Rapid Antigen Tests for Confirmed COVID-19 Cases.” Covid-19 Modelling Aotearoa, August 15, 2022.
Isolating confirmed cases while infectious is an important way of reducing transmission of infectious diseases. The infectious period of most diseases varies between people, therefore matching this period to an ideal isolation period is difficult. Doing so requires balancing the impact that isolation has on individuals and the community with the risk of avoidable onward infections if people are released from isolation while still infectious. Rapid antigen tests (RATs) have been suggested as a way to help identify cases who are still infectious and to better target isolation requirements. We extended the approach used by the UKHSA to investigate the impact of different isolation periods and test-to-release conditions for SARS-CoV-2.
We produced two reports which quantified a range of output metrics including hours infectious in the community and hours of excess isolation for different case isolation policies, including with the use of test-to-release (TTR). We found that we could use TTR to make people safe and require less cost and disruption to the economy from excess isolation.
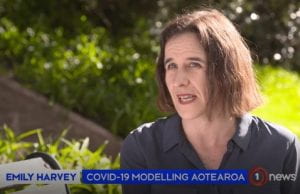
Dr Emily Harvey speaks to 1 News
We published the code for these simulations, making it possible for anyone easily compare and evaluate different case isolation policy options. This ensured that calculations were transparent, assumptions were clear, and the research was reproducible. The work resulted in significant media interest. Newsroom’s Marc Daalder wrote an article about different isolation options and their consequences. It included interactive visualisations of model results, built from our publicly released code, to illustrate different scenarios covered in the article.
Journal Articles
- Binny, Rachelle N, Patricia Priest, Nigel P French, Matthew Parry, Audrey Lustig, Shaun C Hendy, Oliver J Maclaren, et al. “Sensitivity of Reverse Transcription Polymerase Chain Reaction Tests for Severe Acute Respiratory Syndrome Coronavirus 2 Through Time.” The Journal of Infectious Diseases 227, no. 1 (January 1, 2023): 9–17. https://doi.org/10.1093/infdis/jiac317.
- Binny, Rachelle N., Audrey Lustig, Shaun C. Hendy, Oliver J. Maclaren, Kannan M. Ridings, Giorgia Vattiato, and Michael J. Plank. “Real-Time Estimation of the Effective Reproduction Number of SARS-CoV-2 in Aotearoa New Zealand.” PeerJ 10 (October 17, 2022): e14119. https://doi.org/10.7717/peerj.14119.
- Binny, Rachelle N., Michael G. Baker, Shaun C. Hendy, Alex James, Audrey Lustig, Michael J. Plank, Kannan M. Ridings, and Nicholas Steyn. “Early Intervention Is the Key to Success in COVID-19 Control.” Royal Society Open Science 8, no. 11 (November 17, 2021): 210488. https://doi.org/10.1098/rsos.210488.
- Harvey, Emily P., Joel A. Trent, Frank Mackenzie, Steven M. Turnbull, and Dion R. J. O’Neale. “Calculating Incidence of Influenza-like and COVID-like Symptoms from Flutracking Participatory Survey Data.” MethodsX 9 (January 1, 2022): 101820. https://doi.org/10.1016/j.mex.2022.101820.
- Hendy, Shaun, Nicholas Steyn, Alex James, Michael J. Plank, Kate Hannah, Rachelle N. Binny, and Audrey Lustig. “Mathematical Modelling to Inform New Zealand’s COVID-19 Response.” Journal of the Royal Society of New Zealand 51, no. sup1 (May 31, 2021): S86–106. https://doi.org/10.1080/03036758.2021.1876111.
- James, A, M J Plank, R N Binny, A Lustig, K Hannah, S C Hendy, and N Steyn. “A Structured Model for COVID-19 Spread: Modelling Age and Healthcare Inequities.” Mathematical Medicine and Biology: A Journal of the IMA 38, no. 3 (August 15, 2021): 299–313. https://doi.org/10.1093/imammb/dqab006.
- James, A., M. J. Plank, S. Hendy, R. Binny, A. Lustig, N. Steyn, A. Nesdale, and A. Verrall. “Successful Contact Tracing Systems for COVID-19 Rely on Effective Quarantine and Isolation.” PLOS ONE 16, no. 6 (March 6, 2021): e0252499. https://doi.org/10.1371/journal.pone.0252499.
- James, Alex, Michael J. Plank, Shaun Hendy, Rachelle N. Binny, Audrey Lustig, and Nic Steyn. “Model-Free Estimation of COVID-19 Transmission Dynamics from a Complete Outbreak.” PLOS ONE 16, no. 3 (March 24, 2021): e0238800. https://doi.org/10.1371/journal.pone.0238800.
- Lustig, Audrey, Giorgia Vattiato, Oliver Maclaren, Leighton M. Watson, Samik Datta, and Michael J. Plank. “Modelling the Impact of the Omicron BA.5 Subvariant in New Zealand.” Journal of The Royal Society Interface 20, no. 199 (February 2023): 20220698. https://doi.org/10.1098/rsif.2022.0698.
- Plank, Michael J, Alex James, Audrey Lustig, Nicholas Steyn, Rachelle N Binny, and Shaun C Hendy. “Potential Reduction in Transmission of COVID-19 by Digital Contact Tracing Systems: A Modelling Study.” Mathematical Medicine and Biology: A Journal of the IMA 39, no. 2 (June 11, 2022): 156–68. https://doi.org/10.1093/imammb/dqac002.
- Plank, Michael J., Rachelle N. Binny, Shaun C. Hendy, Audrey Lustig, and Kannan Ridings. “Vaccination and Testing of the Border Workforce for COVID-19 and Risk of Community Outbreaks: A Modelling Study.” Royal Society Open Science 8, no. 9 (September 29, 2021): 210686. https://doi.org/10.1098/rsos.210686.
- Plank, Michael J., Shaun C. Hendy, Rachelle N. Binny, Giorgia Vattiato, Audrey Lustig, and Oliver J. Maclaren. “Using Mechanistic Model-Based Inference to Understand and Project Epidemic Dynamics with Time-Varying Contact and Vaccination Rates.” Scientific Reports 12, no. 1 (November 28, 2022): 20451. https://doi.org/10.1038/s41598-022-25018-3.
- Steyn, Nicholas, Audrey Lustig, Shaun C. Hendy, Rachelle N. Binny, and Michael J. Plank. “Effect of Vaccination, Border Testing, and Quarantine Requirements on the Risk of COVID-19 in New Zealand: A Modelling Study.” Infectious Disease Modelling 7, no. 1 (March 2022): 184–98. https://doi.org/10.1016/j.idm.2021.12.006.
- Steyn, Nicholas, Michael J. Plank, Alex James, Rachelle N. Binny, Shaun C. Hendy, and Audrey Lustig. “Managing the Risk of a COVID-19 Outbreak from Border Arrivals.” Journal of The Royal Society Interface 18, no. 177 (April 21, 2021): 20210063. https://doi.org/10.1098/rsif.2021.0063.
- Steyn, Nicholas, Michael J. Plank, Rachelle N. Binny, Shaun C. Hendy, Audrey Lustig, and Kannan Ridings. “A COVID-19 Vaccination Model for Aotearoa New Zealand.” Scientific Reports 12, no. 1 (February 17, 2022): 2720. https://doi.org/10.1038/s41598-022-06707-5.
- Steyn, Nicholas, Rachelle N. Binny, Kate Hannah, Shaun C. Hendy, Alex James, Tahu Kukutai, Audrey Lustig, et al. “Estimated Inequities in COVID-19 Infection Fatality Rates by Ethnicity for Aotearoa New Zealand.” New Zealand Medical Journal Vol 133, no. No 1521 (April 24, 2020). https://doi.org/10.1101/2020.04.20.20073437.
- Steyn, Nicholas, Rachelle N Binny, Kate Hannah, Shaun C Hendy, Alex James, Audrey Lustig, Kannan Ridings, Michael J Plank, and Andrew Sporle. “Māori and Pacific People in New Zealand Have a Higher Risk of Hospitalisation for COVID-19” New Zealand Medical Journal Vol 134, no. 1538 (2021).
- Turnbull, S. M., M. Hobbs, L. Gray, E. P. Harvey, W. M. L. Scarrold, and D. R. J. O’Neale. “Investigating the Transmission Risk of Infectious Disease Outbreaks through the Aotearoa Co-Incidence Network (ACN): A Population-Based Study.” The Lancet Regional Health – Western Pacific 20 (March 1, 2022). https://doi.org/10.1016/j.lanwpc.2021.100351.
- Vattiato, Giorgia, Audrey Lustig, Oliver J. Maclaren, and Michael J. Plank. “Modelling the Dynamics of Infection, Waning of Immunity and Re-Infection with the Omicron Variant of SARS-CoV-2 in Aotearoa New Zealand.” Epidemics 41 (December 1, 2022): 100657. https://doi.org/10.1016/j.epidem.2022.100657.
- Vattiato, Giorgia, Audrey Lustig, Oliver Maclaren, Rachelle N. Binny, Shaun C. Hendy, Emily Harvey, Dion O’Neale, and Michael J. Plank. “Modelling Aotearoa New Zealand’s COVID-19 Protection Framework and the Transition Away from the Elimination Strategy.” Royal Society Open Science 10, no. 2 (February 2023): 220766. https://doi.org/10.1098/rsos.220766.
- Vattiato, Giorgia, Oliver Maclaren, Audrey Lustig, Rachelle N. Binny, Shaun C. Hendy, and Michael J. Plank. “An Assessment of the Potential Impact of the Omicron Variant of SARS-CoV-2 in Aotearoa New Zealand.” Infectious Disease Modelling 7, no. 2 (June 1, 2022): 94–105. https://doi.org/10.1016/j.idm.2022.04.002.
Pre-prints
- Binny, Rachelle N., Audrey Lustig, Ann Brower, Shaun C. Hendy, Alex James, Matthew Parry, Michael J. Plank, and Nicholas Steyn. “Effective Reproduction Number for COVID-19 in Aotearoa New Zealand.” medRxiv, August 11, 2020. https://doi.org/10.1101/2020.08.10.20172320.
- Binny, Rachelle N., Shaun C. Hendy, Alex James, Audrey Lustig, Michael J. Plank, and Nicholas Steyn. “Effect of Alert Level 4 on Reff: Review of International COVID-19 Cases.” medRxiv, May 6, 2020. https://doi.org/10.1101/2020.04.30.20086934.
- Binny, Rachelle N., Shaun C. Hendy, Alex James, Audrey Lustig, Michael J. Plank, and Nicholas Steyn. “Probability of Elimination for COVID-19 in Aotearoa New Zealand.” medRxiv, August 11, 2020. https://doi.org/10.1101/2020.08.10.20172361.
- James, Alex, Shaun C. Hendy, Michael J. Plank, and Nicholas Steyn. “Suppression and Mitigation Strategies for Control of COVID-19 in New Zealand.” medRxiv, March 30, 2020. https://doi.org/10.1101/2020.03.26.20044677.
- Musoke, Nathan, Emily Kendall, Mateja Gosenca, Lillian Guo, Lerh Feng Low, Angela Xue, and Richard Easther. “Commuter Count: Inferring Travel Patterns from Location Data.” arXiv, March 30, 2023. https://doi.org/10.48550/arXiv.2303.17758.
- Watson, Leighton M., Michael J. Plank, Bridget A. Armstrong, Joanne R. Chapman, Joanne Hewitt, Helen Morris, Alvaro Orsi, Michael Bunce, Christl A. Donnelly, and Nicholas Steyn. “Improving Estimates of Epidemiological Quantities by Combining Reported Cases with Wastewater Data: A Statistical Framework with Applications to COVID-19 in Aotearoa New Zealand.” medRxiv, August 16, 2023. https://doi.org/10.1101/2023.08.14.23294060.
Reports, Articles, and Advice Published on covid19modelling.ac.nz
- Arnold, Richard, Rachelle N Binny, Thomas Lumley, Audrey Lustig, Matthew Parry, and Michael Plank. “Estimating COVID19 Border Arrival Risk in New Zealand.” Covid-19 Modelling Aotearoa, May 17, 2022. https://covid19modelling.blogs.auckland.ac.nz/estimating-covid-19-border-arrival-risk-in-aotearoa-new-zealand/.
- Datta, Samik, Emily Harvey, Dion O’Neale, Ella Priest Forsyth, and Giorgia Vattiato. “Modelling Scenarios for Changing COVID-19 Isolating Behaviour and Transmission.” Covid-19 Modelling Aotearoa, June 26, 2023. https://covid19modelling.blogs.auckland.ac.nz/modelling-scenarios-for-changing-covid-19-isolating-behaviour-and-transmission/.
- Datta, Samik, James Gilmour, Emily Harvey, Oliver J Maclaren, Dion R J O’Neale, Frankie Patten-Elliott, Michael J Plank, Ella Priest-Forsyth, Steven Turnbull, and David Wu. “Modelling the Effect of Changes to the COVID-19 Case Isolation Policy.” Covid-19 Modelling Aotearoa, 2023. https://covid19modelling.blogs.auckland.ac.nz/modelling-the-effect-of-changes-to-the-covid-19-case-isolation-policy/.
- Datta, Samik, Oliver Maclaren, Michael J Plank, and Giorgia Vattiato. “Modelling the Effect of Alternative Covid-19 Isolation Policy Options.” Covid-19 Modelling Aotearoa, August 17, 2023. https://www.covid19modelling.ac.nz/modelling-the-effect-of-covid-19-isolation-policy-options/.
- Gilmour, James, Emily Harvey, Dion O’Neale, and Steven Turnbull. “‘Phased Transition’ to Phase Transition: The Network Consequences of Reconnecting.” Covid-19 Modelling Aotearoa, October 9, 2021. https://covid19modelling.blogs.auckland.ac.nz/phased-transition-to-phase-transition/.
- Gilmour, James, Emily Harvey, Frank Mackenzie, Oliver Maclaren, Frankie Patten-Elliott, Steven Turnbull, and David Wu. “Impact of Wastewater Testing Results on Preliminary Estimates of COVID-19 Community Transmission as of 18 August 202.” Report to government. Covid-19 Modelling Aotearoa, September 15, 2021. https://covid19modelling.blogs.auckland.ac.nz/contagion-network-modelling-in-the-first-weeks-of-the-august-2021-outbreak/.
- Gilmour, James, Emily Harvey, Joshua Looker, Frank Mackenzie, and Steven Turnbull. “Inter-Regional Movement and Contagion Risk Analysis August 202.” Report to government. Covid-19 Modelling Aotearoa, August 14, 2021. https://covid19modelling.blogs.auckland.ac.nz/inter-regional-movement-and-contagion-risk/.
- Gilmour, James, Emily Harvey, Joshua Looker, Frank Mackenzie, Dion O’Neale, Frankie Patten-Elliott, Joel Trent, Steven Turnbull, and David Wu. “Estimates of Effects on Changing Alert Levels for the August 2021 Outbreak.” Report to government. Covid-19 Modelling Aotearoa, September 9, 2021. https://covid19modelling.blogs.auckland.ac.nz/estimates-of-effects-of-changing-alert-levels-for-the-august-2021-outbreak/.
- Gilmour, James, Emily Harvey, Joshua Looker, Frank Mackenzie, Dion O’Neale, Frankie Patten-Elliott, Joel Trent, and David Wu. “Modelling Estimates of Expected Size: The August 2021 COVID-19 Outbreak in Aotearoa.” Report to government. Covid-19 Modelling Aotearoa, September 15, 2021. https://covid19modelling.blogs.auckland.ac.nz/contagion-network-modelling-in-the-first-weeks-of-the-august-2021-outbreak/.
- Gilmour, James, Emily Harvey, Oliver Maclaren, Dion O’Neale, Steven Turnbull, and David Wu. “Preliminary Estimates of Hospitalisation Numbers for the August 2021 Outbreak, Assuming We Stay in Alert Level 4.” Covid-19 Modelling Aotearoa, October 15, 2021. https://covid19modelling.blogs.auckland.ac.nz/preliminary-estimates-of-hospitalisation-numbers/.
- Harvey, Emily P, Joshua Looker, Dion R J O’Neale, Michael Plank, Ella Priest, and Joel Trent. “Quantifying the Impact of Isolation Period and the Use of Rapid Antigen Tests for Confirmed COVID-19 Cases.” Covid-19 Modelling Aotearoa, August 15, 2022. https://covid19modelling.blogs.auckland.ac.nz/quantifying-the-impact-of-isolation-period/.
- Harvey, Emily P, Matt Hobbs, Joshua Looker, Dion R J O’Neale, and Steven Martin Turnbull. “Exploring COVID-19 Transmission Risk and Vulnerability Through the Aotearoa Co-Incidence Network (ACN).” Technical Report. Covid-19 Modelling Aotearoa, February 9, 2021. https://covid19modelling.blogs.auckland.ac.nz/aotearoa-co-incidence-network/.
- Harvey, Emily, and Dion O’Neale. “Estimates of the Effect and Impact of Different Case Isolation Policies.” Advice to govt. Covid-19 Modelling Aotearoa, August 17, 2023. https://www.covid19modelling.ac.nz/modelling-the-effect-of-covid-19-isolation-policy-options/.
- Harvey, Emily, Frank Mackenzie, Dion O’Neale, and Steven Turnbull. “Estimates of New Onset of Covid-like Illness, Symptomatic Population, and Testing Rates.” Covid-19 Modelling Aotearoa, February 9, 2021. https://covid19modelling.blogs.auckland.ac.nz/estimates-of-new-onset-of-covid-like-illness-symptomatic-population-and-testing-rates/.
- Harvey, Emily, Frank Mackenzie, Dion O’Neale, and Steven Turnbull. “FluTracking Incidence Calculation Methods.” Technical Report. Covid-19 Modelling Aotearoa, September 20, 2021. https://covid19modelling.blogs.auckland.ac.nz/flutracking-incidence-calculation-methods/.
- Harvey, Emily, Oliver Maclaren, Dion O’Neale, Adrian Ortiz-Cervantes, Steven Turnbull, Demival Vasques Filho, and David Wu. “Network-Based Simulations of Re-Emergence and Spread of COVID-19 in Aotearoa New Zealand.” Technical Report. Te Pūnaha Matatini, October 19, 2020. https://covid19modelling.blogs.auckland.ac.nz/simulations-of-re-emergence-and-spread/.
- Harvey, Emily, Oliver Maclaren, Dion O’Neale, Frankie Patten-Elliott, and David Wu. “Alert Level 2.5 Is Insufficient for Suppression or Elimination of COVID-19 Community Outbreak.” Technical Report. Covid-19 Modelling Aotearoa, February 15, 2021. https://covid19modelling.blogs.auckland.ac.nz/network-modelling-trilogy/.
- Harvey, Emily, Oliver Maclaren, Dion O’Neale, Frankie Patten-Elliott, and David Wu. “Contagion Network Modelling of Effectiveness for a Range of Non-Pharmaceutical Interventions for COVID-19 Elimination in Aotearoa New Zealand.” Technical Report. Covid-19 Modelling Aotearoa, July 19, 2021. https://covid19modelling.blogs.auckland.ac.nz/network-modelling-trilogy/.
- Harvey, Emily, Oliver Maclaren, Dion O’Neale, Frankie Patten-Elliott, and David Wu. “Network Modelling of Elimination Strategy Pillars: Prepare for It, Stamp It Out.” Technical Report. Covid-19 Modelling Aotearoa, July 20, 2021. https://covid19modelling.blogs.auckland.ac.nz/network-modelling-trilogy/.
- Hendy, Shaun C., Nicholas Steyn, Michael J. Plank, and Rachelle N. Binny. “Technical Report: Modelling the Potential Spread of COVID-19 during the August 2021 Outbreak.” Technical Report. Covid-19 Modelling Aotearoa, August 28, 2021. https://covid19modelling.blogs.auckland.ac.nz/modelling-the-potential-spread-of-covid-19-during-the-august-2021-outbreak/.
- Hendy, Shaun C., Rachelle N. Binny, Nicholas Steyn, and Michael J. Plank. “Technical Report: Update to Modelling 7 September 2021.” Technical Report. Covid-19 Modelling Aotearoa, September 7, 2021. https://covid19modelling.blogs.auckland.ac.nz/modelling-the-potential-spread-of-covid-19-during-the-august-2021-outbreak/.
- James, Alex, Audrey Lustig, Kannan Ridings, Michael J Plank, Rachelle N Binny, and Nicholas Steyn. “Modelling Support for the Continued Elimination Strategy.” Technical Report. Te Pūnaha Matatini, August 12, 2020. https://covid19modelling.blogs.auckland.ac.nz/modelling-support-for-the-continued-elimination-strategy/.
- James, Alex, Michael J Plank, Rachelle N Binny, Shaun C Hendy, and Audrey Lustig. “Summary of Advice to Cabinet on Auckland’s August 2020 COVID-19 Outbreak.” Advice to govt. Te Pūnaha Matatini, October 14, 2020. https://covid19modelling.blogs.auckland.ac.nz/aucklands-august-outbreak/.
- James, Alex, Rachelle N. Binny, Shaun C. Hendy, Audrey Lustig, Nicholas Steyn, and Michael J. Plank. “Economic Comparison of the Use of Alert Levels 3 and 4 in Eliminating the Auckland August Outbreak: A Cost-Effectiveness Analysis.” Technical Report. Te Pūnaha Matatini, October 21, 2020. https://covid19modelling.blogs.auckland.ac.nz/economic-comparison-of-the-use-of-alert-levels-3-and-4/.
- O’Neale, Dion R J, Emily P Harvey, and Ella Priest-Forsyth. “Quantifying the Effect of a Change in Case Isolation Settings.” Covid-19 Modelling Aotearoa, April 20, 2023. https://covid19modelling.blogs.auckland.ac.nz/quantifying-the-effect-of-a-change-in-case-isolation-settings/.
- O’Neale, Dion R J. “Contagion Network Modelling in the First Weeks of the August 2021 Outbreak.” Report to government. Covid-19 Modelling Aotearoa, July 9, 2021. https://covid19modelling.blogs.auckland.ac.nz/contagion-network-modelling-in-the-first-weeks-of-the-august-2021-outbreak/.
- Plank, Michael J, Rachelle N Binny, Shaun C Hendy, Audrey Lustig, and Alex James. “Effective Reproduction Number and Likelihood of Cases Outside Auckland.” Technical Report. Te Pūnaha Matatini, September 15, 2020. https://covid19modelling.blogs.auckland.ac.nz/likelihood-of-covid-19-spreading-outside-auckland/.
- Plank, Michael J., Shaun C. Hendy, Oliver J. Maclaren, and Rachelle N. Binny. “Technical Report: Modelling the August 2021 COVID-19 Outbreak in New Zealand.” Technical Report. Covid-19 Modelling Aotearoa, October 18, 2021. https://covid19modelling.blogs.auckland.ac.nz/modelling-the-august-2021-covid-19-outbreak/.
- Steyn, Nicholas, Michael J. Plank, and Shaun C. Hendy. “Modelling to Support a Future COVID-19 Strategy for Aotearoa New Zealand.” Covid-19 Modelling Aotearoa, September 23, 2021. https://covid19modelling.blogs.auckland.ac.nz/modelling-to-support-a-future-covid-19-strategy/.
- Vattiato, Giorgia, Oliver Maclaren, Audrey Lustig, Rachelle N Binny, Shaun C Hendy, and Michael J Plank. “A Preliminary Assessment of the Potential Impact of the Omicron Variant of SARS-CoV-2 in Aotearoa New Zealand.” Covid-19 Modelling Aotearoa, January 23, 2022. https://covid19modelling.blogs.auckland.ac.nz/a-preliminary-assessment-of-the-potential-impact-of-the-omicron-variant/.
- Vattiato, Giorgia, Samik Datta, Oliver J Maclaren, Audrey Lustig, and Michael J Plank. “Modelling Covid-19 Dynamics in New Zealand August 2022 to February 2023.” Technical Report. Covid-19 Modelling Aotearoa, February 3, 2023. https://covid19modelling.blogs.auckland.ac.nz/modelling-covid-19-dynamics-in-new-zealand-august-2022-to-february-2023/.
Software
- The Populated Aotearoa Interaction Network (PAIN) – an extensive software library written in R and Python for processing demographic data from a range of sources and using the resulting synthetic population to build a bipartite interaction network for Aotearoa (or sub-regions). PAIN and CoBiN together comprise the Network Contagion Model described above. PAIN requires inputs of a number of custom data tables in order to characterise the approx 5 million individuals in Aotearoa along with their interaction contexts (dwellings, workplaces, schools, and “community” events). A version of the library suitable for public release is in progress. Access to the non-public code repository has been provided to analysts at NZ MoH.
- Contagion on a Bipartite Network (CoBiN) – a library written in the Python programming language for simulating disease spread on a bipartite network, where individuals are linked to explicit interactions contexts. Requires a network built from the PAIN software as input, though sample networks are included with the code via a figshare link.
- code: https://gitlab.com/cma-public-projects/cobin
- documentation: https://cma-public-projects.gitlab.io/cobin/
- Stochastic Simulations for Managing Isolation & Quarantine - a package written in the Julia programming language for fast stochastic simulation of the direct impact of different case isolation and contact quarantine scenarios, including test-to-release. Calibrated to international literature for viral load and test sensitivity and to case data from Aotearoa for contact testing behaviour.
- Flutracking – a library of analytic tools for calculating robust incidence rates for respiratory disease in Aotearoa from weekly Flutracking survey data. Includes methods to adjust for response bias, population re-weighting, and calculating confidence intervals. Code is written in the R programming language; an older version of the public dash-board, using R Shiny is available.
- dashboard: https://siicc.covid19modelling.ac.nz/
- analytic tools & code: https://gitlab.com/cma-public-projects/flutracking-methods-article
- The Aotearoa Co-incidence Network (ACN) – this repository consists of code (R and SQL) that can be run inside the Statistics NZ Integrated Data Infrastructure (IDI) to produce a table of counts for the number of connections between area units when dwellings are linked through an interaction at a shared school or workplace. The repository also includes code (written in R) for analysis and visualisation of the aggregated data after extraction from the IDI. This includes methods for estimating transmission risk and its correlation with age and vulnerability.
- interactive visualisation: https://stur600.shinyapps.io/aotearoa-coincidence-network/
- analytic tools & code: https://gitlab.com/cma-public-projects/aotearoa-connection-network/

Dr Dion O'Neale speaking on TVNZ's Breakfast.
Our researchers are sought-after and trusted experts. Over the full course of the programme, they’ve collectively fielded well over a thousand media requests, resulting in quotes across a vast range of local and international media including RNZ, NZ Herald, Stuff.co.nz, The Project, NewstalkZB, 1 News, NewsHub, Newsroom, The Guardian, The BBC, Te Hiku Media, and assorted regional newspapers. They have also written widely syndicated articles for The Conversation and The Spinoff which have seen a readership in the hundreds of thousands. Our team has raised the profile of mathematical modelling, and has worked hard to communicate our findings with the public, to help Aotearoa better understand disease spread and how to keep themselves safer.
Editorial highlights
Plank, M., Shearer, F., McCaw, J., Wood, J. With COVID now endemic, modelling suggests targeted protection will be more effective than blanket measures. The Conversation, August 24 2023.
Baker, M., Welch, D., & O’Neale, D. With COVID on the rise again, here are some simple steps to help us socialise safely during the holidays. The Conversation, December 20, 2022.
Plank, M., Geoghegan, J., Welch, D. With most mandatory public health measures gone, is New Zealand well prepared for the next COVID wave? The Conversation, September 15 2022.
Plank, M., Lustig, A., Welch, D., Vattiato, G. A new Omicron wave is upon New Zealand, with older people now most at risk – here’s what to expect. The Conversation, July 7 2023.
Plank, M., O’Neale, D., & Harvey, E. As New Zealand relaxes restrictions, here’s what we can still do to limit COVID infections. The Conversation. March 23, 2022.
O’Neale, D. NZ’s confirmed COVID case numbers are rising fast, but total infections are likely much higher – here’s why. The Conversation. February 25, 2022.
O'Neale, D., Harvey, E., Sporle, A., & Turnbull, S. As Aucklanders anticipate holiday trips, Māori leaders ask people to stay away from regions with lower vaccination rates. The Conversation. November 30, 2021.
O'Neale, D., Harvey, E., Sporle, A., & Turnbull, S. How to lower your Covid risk these summer holidays. The Spinoff, November 30, 2021.
O'Neale, D., Harvey, E., Gilmour, J., & Turnbull, S. New Zealanders are super-connected. When restrictions lift in Auckland, it won’t take much to amplify Delta’s spread. The Conversation, October 26, 2021.
O'Neale, D., Harvey, E., Gilmour, J., & Turnbull, S. We built a model that shows how small rule changes can fuel deltas spread. The Spinoff, October 26, 2021.